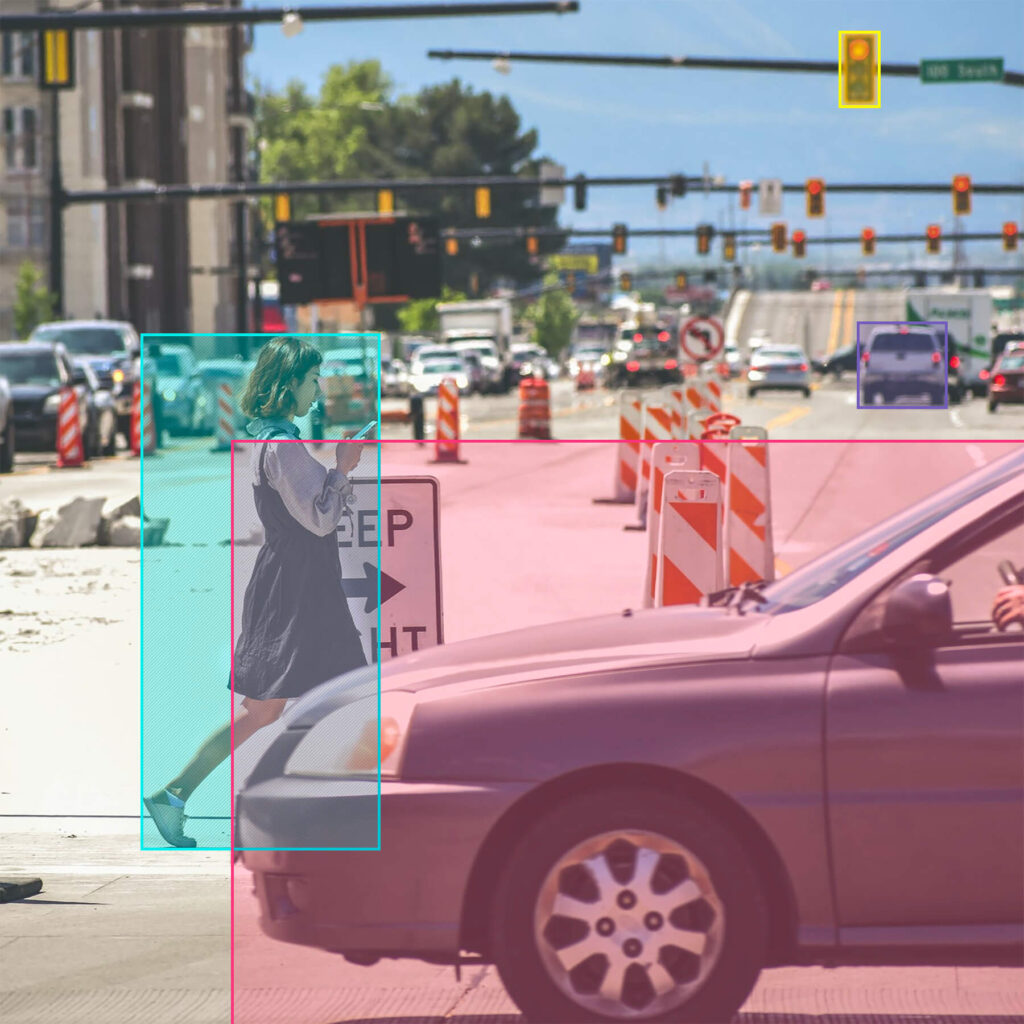
Automotive Industries interview with Duncan Curtis, SVP of Product & Technology at Sama
In the following interview with Automotive Industries, Duncan Curtis, SVP of Product & Technology at Sama, delves into the complexities of annotating medium and long sequences of frames for automotive AI models. Curtis highlights the sheer volume of visual information AI must process to navigate effectively, from other vehicles and road signs to pedestrians and obstacles.
This translates to thousands of frames per mile that need precise annotation, posing significant challenges for model accuracy and responsiveness.
Sama’s innovative solution tackles these challenges by achieving up to a 99% quality rate in annotating both 3D dynamic and static objects, scaling up to 35 million frames in just eight months. Curtis emphasizes the critical role of the Human in the Loop (HITL) approach, blending human expertise with proprietary algorithms. This combination ensures high-quality annotations, particularly in complex scenarios like tracking vehicles that move unpredictably.
The company’s proprietary algorithms excel in handling predictable data, such as road lines, while human annotators focus on more variable elements. This hybrid approach not only enhances accuracy but also mitigates the risk of costly retraining due to data errors. Curtis underscores that their expert workforce and stringent quality controls, including project-specific training and an AutoQA process, are key to maintaining their high standards.
Sama’s social impact focus further sets it apart, providing living wages and benefits to its employees, many of whom are entering the digital economy for the first time. This ethical approach aligns with their commitment to responsible AI development.
Curtis also discusses how Sama’s efficient, high-quality annotation services contribute to faster rollout and reduced costs for clients, which include 80% of the top OEM and Tier 1 suppliers. This efficiency is crucial as ADAS systems become more standard and demand continuous model improvement.
Sama is poised to further refine its processes, aiming to push the boundaries of their technology to meet the evolving needs of automotive applications. Curtis’s enthusiasm reflects Sama’s commitment to innovation and excellence in the fast-paced world of automotive AI.
Â
Automotive Industries interview with Duncan Curtis, SVP of Product & Technology at Sama
Automotive Industries: Hi Duncan, what are the key challenges in annotating medium and long sequences of frames for automotive AI models, and how does Sama’s new solution address these challenges?
Curtis: Think about how much you have to take in and process visually when you’re driving a car – other vehicles ranging from motorcycles to semi-trucks, the lines on the road, pedestrians, potholes, any signs or lights, and that’s just the start.
Â
For an AI model to successfully drive, it must be able to process, identify, and appropriately respond to the hundreds of inputs it’s receiving. Just 10 miles of driving can represent thousands or even hundreds of thousands of frames that all need to be annotated accurately and consistently, or the model may fail to respond correctly. That is before you get into the number of camera angles and other complicating factors.
Â
 At Sama, we’ve developed a solution that provides up to a 99% quality rate for both 3D dynamic and static objects at the scale needed for these kinds of sequences – 35 million frames in 8 months.
Automotive Industries: How does Sama ensure the quality and consistency of annotations across multiple camera angles and 3D perspectives in automotive data?
Curtis: At Sama, we embrace the Human in the Loop (HITL) approach in everything we do. While I can’t share all of the details as to how we’ve achieved this high of a quality rate, I can say that human feedback is essential to ensuring quality. We have an expert workforce for each of the projects we undertake and combine human review and annotation with our proprietary algorithms to constantly deliver quality and accuracy.
Automotive Industries: Can you explain how Sama’s proprietary algorithms and human feedback processes work together to optimize the annotation process?
Duncan Curtis on challenges in 3D LiDAR annotations and data sets
Curtis: Our algorithms are great at helping us quickly annotate the more stable or predictable parts of automotive data – like where the lines are on the road, for example. But when it comes to tracking a car, which may go in and out of the camera’s view or change position, humans are more equipped to annotate the data correctly and consistently.
Automotive Industries: How does Sama’s solution help reduce the total cost of ownership for automotive data annotation for OEMs and Tier 1 suppliers?
Curtis: Developing models is expensive. There’s no getting around that. But costs can balloon rapidly when a model has to go through multiple rounds of retraining because of issues in the data, such as improperly annotated objects or inconsistencies. As a result, an inexperienced data annotator and model evaluator can be very costly to the customer, as they then need to perform more sampling work to assess accuracy. Rework, even if it’s free to the customer, costs time in the form of delayed launches. The majority of our customers come to us because we have the kind of deep experience to get a project right the first time.
Automotive Industries: What specific training and quality control measures does Sama implement to ensure high-quality annotations for complex 3D workflows?
Curtis: We pride ourselves on our industry-leading customer acceptance rates. In our experience, we’ve learned that specific training for each project is the best method to ensure our annotators are all equipped for their work. For example, the standards of what counts as a good annotation can be a little different from project to project. By completing training before each project, annotators have the right standards fresh in their minds. We also have an AutoQA process that identifies logical fallacies and surfaces them to annotators before the QA process even begins. That can save our teams multiple hours each day.
Automotive Industries: How does Sama’s approach to data annotation contribute to creating a more ethical and responsible AI supply chain for the automotive industry?
Curtis: Sama is a social impact-focused organization and a public benefit corporation. We pay our full-time, in-house employees living wages and provide benefits – for many of them, this is their first formal job and work in the digital economy. For AI to be produced responsibly, everyone in the supply chain needs to be thinking about how they can treat workers fairly while following all regulations and standards they need to. We also offer comprehensive model evaluation services because we believe that validating your model before it’s deployed is a critical aspect of responsible development.
Automotive Industries: What are some of the key factors that differentiate Sama’s data annotation services from other providers in the automotive industry?
Curtis: One of our biggest differentiators is in our quality work without sacrificing scale. Even in a complex 3D workflow project, we’re able to deliver up to 10 million annotated shapes a day – again at that 99% rate. Another standout is our experience. Our employees are experts in these kinds of projects and regularly refresh themselves on the best practices in complex tasks like 3D multi-sensor annotations. We support 80% of the top OEM and Tier 1 suppliers in the world. We are working with them on the cutting edge of ADAS and automotive AI.
Automotive Industries: How has Sama’s annotation work impacted the development and performance of advanced driver assistance systems (ADAS) and autonomous vehicle models for your clients?
Curtis: Obviously, the reduced total cost of ownership is a major factor for our clients. ADAS systems are becoming increasingly standard, meaning that companies must continually improve their models or add more annotated data. The faster that these models can be rolled out, the better it is. How much sampling a customer has to do and the resulting rework are two major factors in determining how long it takes for a model to get to market. The less sampling, the faster the rollout.
Automotive Industries: Looking forward, what are the next steps for Sama in scaling its data annotation capabilities for even longer sequences of frames in automotive applications?
Curtis: We are always working to fine-tune our processes. We have a five-step process already in place when it comes to annotating these kinds of complex sequences, and each project provides us with a deeper understanding of what our customers need and how we can deliver that at the quality they require in the timeframe they need. Stay tuned for more – we are excited to see how far we can push our technology.
Â
Â
Sama Resources:
Sama main page on ADAS & Autonomous Vehicle Solutions
Wendy Gonzalez Forbes Councils Member article from 2022: Three Ways AI Is Impacting The Automobile Industry
How AI Happens Podcast w/Mercedes-Benz Executive Manager for AI Alex Dogariu
More Stories
Cybord TCI – The Future of Manufacturing Integrity
Flexible Magna Manufacturing Solutions: The Key to Success in the Automotive Industry
DuPont technology helps improve EV battery durability and performance