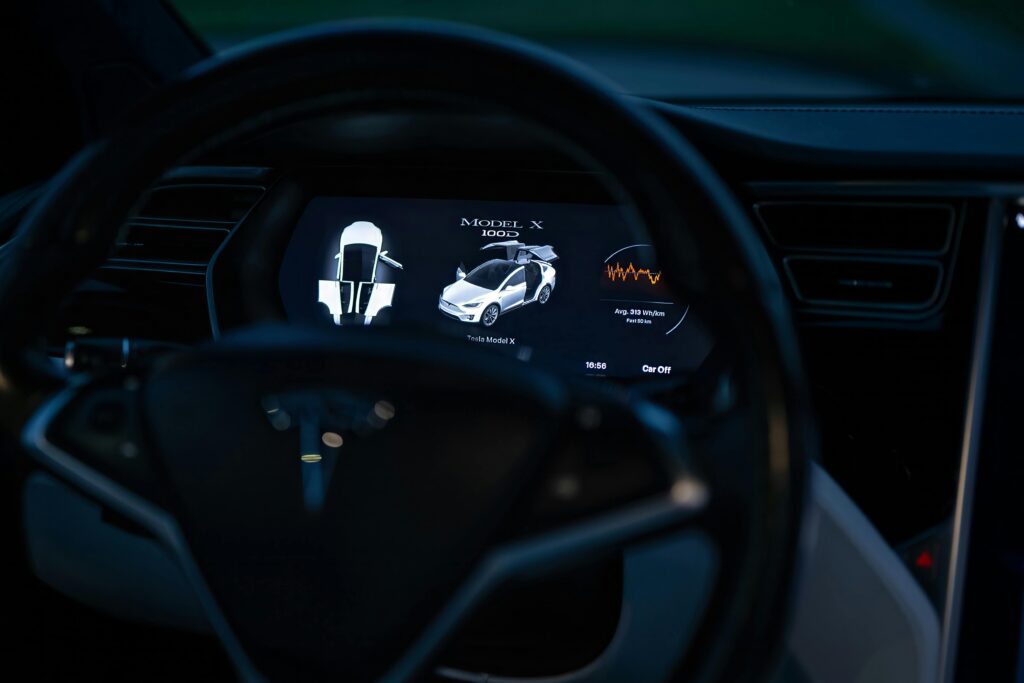
Autonomous vehicles are no longer a distant concept. With each passing year, the technology behind self-driving cars becomes more refined, more integrated, and more capable. According to Statista, around 60% of all cars sold globally will have level 2 autonomy. They are expected to dominate by 2030, with level 3 and level 4 autonomous vehicles to account for 8% of new car sales.
Among the critical elements enabling this shift is sensor fusion. It is the process of combining inputs from multiple sensors to create a comprehensive and real-time understanding of the driving environment. This fusion is crucial for the safe and efficient operation of autonomous systems, especially as vehicles move toward higher levels of automation.
While sensors have individually improved, the power of sensor fusion lies in their coordination. Radar, cameras, LiDAR, ultrasonic sensors, and IMUs all play a role in helping a vehicle “see” and respond to its surroundings. But making them work together efficiently requires sophisticated algorithms, significant computing power, and a deep understanding of both hardware and software systems.
In this article, we will look at advancements in sensor fusion and how they are facilitating the next generation of autonomous driving systems.
Why Sensor Fusion Matters More Than Ever
Level 1 and Level 2 driver assistance systems rely heavily on single-sensor functions, such as parking assistance or blind spot detection. However, as vehicles move toward full autonomy, sensor redundancy and reliability become essential.
No single sensor can handle every driving scenario. A foggy road may blind a camera, while rain can distort LiDAR readings. Sensor fusion balances these shortcomings, allowing each input to validate or supplement the others.
A Nature Journal study concludes that multi-sensor fusion can help self-driving cars achieve accurate object-tracking in adverse weather. Using a camera, LiDAR, and data together enables advanced segmentation through a Deep Q Network (DQN). It can improve image quality with noise removal, adaptive thresholding, and contrast enhancement.
This layered view of the environment improves not just perception but also prediction and decision-making. For example, tracking a cyclist weaving through traffic requires both spatial accuracy and motion analysis. Sensor fusion enables the system to identify the object correctly and forecast its likely path with greater accuracy than any single sensor.
Are there challenges in calibrating multiple sensors for fusion in real-world driving conditions?
Yes, calibration is a major challenge. Each sensor type operates with different timing, resolutions, and fields of view. Over time, physical vibrations, temperature changes, or minor shifts in sensor placement can cause misalignment. Accurate calibration ensures that data from all sensors is aligned correctly in space and time.
The Skill Gap in Advanced Automotive Systems
With these technological leaps comes the need for a new kind of engineer. One who understands embedded systems, machine learning, signal processing, and system-level integration. Traditional mechanical or electrical engineering degrees might not cover the cross-disciplinary knowledge needed to work on these systems effectively.
Many professionals are turning to continued education to keep up with this shift. Thus, they go for a Master’s in Electrical and Computer Engineering (MSECE) in advanced mobility. According to Kettering University, this is a first-of-its-kind degree specifically designed to meet the demands of advanced mobility.
It focuses on the following essential systems for the future of transportation:
- Integration of electrical and computer systems
- Design of dynamic systems
- Robotics
- Development of advanced mobility applications
Moreover, advancements in education technology have made it possible to pursue this degree online. Someone opting for an online MSECE can get the right skills while continuing to work. This type of program helps build expertise in core areas directly relevant to sensor fusion in autonomous vehicles. The online format also makes it easier to align coursework with current industry trends and personal career goals.
How Sensor Fusion is Evolving
Early sensor fusion models relied on basic data alignment and filtering methods such as Kalman filters or simple weighted averages. However, these methods usually have some limitations. For instance, Kalman filters require domain-specific design changes. Moreover, the technique is less suited to track non-linear motion patterns accurately.
While these methods still play a role, modern vehicles are moving toward machine-learning-based approaches. Neural networks can now interpret data across multiple modalities, enabling better object classification and environmental understanding.
For instance, a deep learning model might combine radar and camera data to distinguish between a pedestrian and a roadside sign. These networks improve over time as more data is collected, making them highly adaptive but also computationally demanding. This has led to a move away from distributed systems and toward centralized computing platforms that can manage high data loads.
A study from OAE Publishing shows that deep learning can facilitate many things besides sensor fusion, such as:
- Driving scene understanding
- Object detection
- Semantic and instance segmentation
- Localization
- Perception using occupancy grid maps
- Path planning and behavior arbitration
- Online vectorized high-definition map construction
How do developers ensure sensor fusion models remain reliable as technology evolves?
Continuous testing, retraining of algorithms, and validation against updated datasets are essential. Developers often use modular software designs to swap outdated components for improved versions without overhauling entire systems. Benchmarking against industry standards also helps maintain consistency and reliability across different vehicle platforms.
The Role of Simulation and Synthetic Data
Real-world driving data is vital, but it’s often not enough. Collecting edge-case scenarios, such as a child running into the street at dusk, is difficult and potentially dangerous. This is where simulation tools and synthetic data come into play. They allow developers to recreate rare or hazardous situations in a controlled environment.
These synthetic inputs can be fed into sensor fusion models to test how well the system responds to unusual or high-risk scenarios. The growing use of simulated environments is helping shorten development cycles and improve the reliability of autonomous systems.
There are many companies using generative AI to create synthetic data for autonomous cars. The core idea is to use available real-world driving data to simulate novel situations. Engineers focus on creating data that is as realistic as possible while offering something new and distinct from the existing real-world data.
How accurate is synthetic data when compared to real-world sensor input?
Synthetic data has improved significantly, especially with the rise of high-fidelity simulation engines and generative AI. While it can’t fully replace real-world testing, it closely mimics sensor behaviors and edge-case conditions. Validation often involves using synthetic data alongside real-world results to ensure consistent system responses.
Sensor fusion stands as one of the most significant enablers of next-generation autonomous driving systems. Its ability to merge inputs from diverse sensors into a cohesive, reliable understanding of the driving environment is not just a technical achievement. It’s a foundational shift in how vehicles operate and respond.
As the industry continues to push toward higher levels of automation, the integration of hardware and software will only deepen. Engineers equipped with a wide range of skills, including those gained through an online MSECE, will be at the forefront of this evolution. Their work will help shape safer, smarter, and more adaptable mobility systems for years to come.
More Stories
Unique Experiences in Miami: What to Do Off the Beaten Path
The Factors That Impact the Outcome of a Car Accident Claim
Distractions: A Common Cause of Accidents and How to Prevent Them